Even though electric vehicles (EVs) are making good progress in meeting consumer expectations, there remain several challenges. No EV can yet top up range as quickly as is the case with replenishing fuel in a fossil-fuelled vehicle, and range and cost still need to improve before electrification gains universal acceptance.
At the core of meeting these challenges lies the speedy development of the lithium-ion battery. Wan Gang, Vice Chairman of China's national advisory body for policymaking, predicts the global market for EV batteries will reach $250 billion by 2030, with demand exceeding 3.5 terawatt hours.
Yet, time-consuming experimentation and discovery processes continue to curb battery research and development.

Accurately predicting the performance of complex, nonlinear systems found in EV lithium-ion batteries is critical for accelerating the development of the technology. However, the number of variables facing researchers makes plotting a clear development path virtually impossible: diverse chemistries, aging mechanisms, significant device variability, and dynamic operating conditions are some of the challenges facing battery developers.
At the same time, optimizing the many design parameters in time-consuming experiments also slows the progress of battery research and development. This manifests in the lengthy selection process and control optimization of materials, cell manufacturing, and operation.
For instance: Even though a key objective in battery development is to maximize battery lifetime, conducting even a single experiment to determine a cell’s lifetime could take months, or even years.
Ordinarily, data would have to be collected over the entire lifecycle of the battery cell for researchers to predict how the cells would perform in the future. It might take months to cycle the battery enough times to generate the data.
On the other hand, using artificial intelligence (AI) and machine learning, trained on historical data generated during earlier development experimentation and trials, researchers can significantly reduce the time it takes to predict the lifetime performance by extrapolating the outcome even while the battery is still at its peak.
Using AI to overcome the challenges and speed up safe, cost-effective EV battery development

The wide parameter spaces and high sampling variability of battery development necessitate a large number of experiments. This is made more complex by the interrelated nature of a battery’s performance. Often an improvement in one area - say, energy density - will come at a cost to another area, like the charge rate.
AI and machine learning methodology can be implemented to efficiently optimize the simultaneous evaluation of a wide array of variables. By applying AI and machine learning, researchers can speed up the complex and time-consuming development process by orders of magnitude, whilst delivering accurate predictions and results.
For example, to overcome consumers’ “range anxiety” StoreDot researchers have been working on an innovative extremely fast charging (XFC) LI-ion battery. However, with very little research data available on fast charging batteries, this R&D operation, with dozens of Ph.D. researchers and engineers, requires many organized scientific experiments, based on a ‘design of experiments’ matrix to test, maximize, and optimize the battery’s performance, safety, and lifetime.
To obtain accurate results researchers have to carry out simultaneous experimentation with the formulation of chemistries for the active electrode materials, electrochemical profiles, production processes, charging protocols, and safety tests amongst many others.
This produces hundreds of concurrent experiments with thousands of slightly different battery cell permutations, in various sizes and capacities. Running these test procedures while recording all the measurements every second from every one of 4000+ independent battery test channels, creates an enormous amount of data.
With the solution being programmed, automated, and cloud-based, thousands of experiments could be conducted every week. However, even at extreme charging rates, the batteries would still need many months of testing to simulate a whole lifetime.
The process had to be even faster. Researchers needed a way to test or predict each battery’s end of life while keeping results transparent and providing the team with valuable data insights learned from the algorithm.
Analyzing the results obtained from the complex set of experiments on diverse subjects is very similar to clinical trials typically conducted on sample sets of patients, which lead the research team to investigate the possibility of applying this methodology in a machine learning algorithm to speed up battery R&D.
This was accomplished by implementing a new agile research methodology that merged the chemical battery’s world with the concept and methodology applied to the patient clinical trials. Kaplan-Meier graphs and methodologies were found to be ideally suited to investigating, learning, and predicting the battery lifetime.
StoreDot applies the Kaplan–Meier AI algorithm for faster and smarter XFC battery R&D
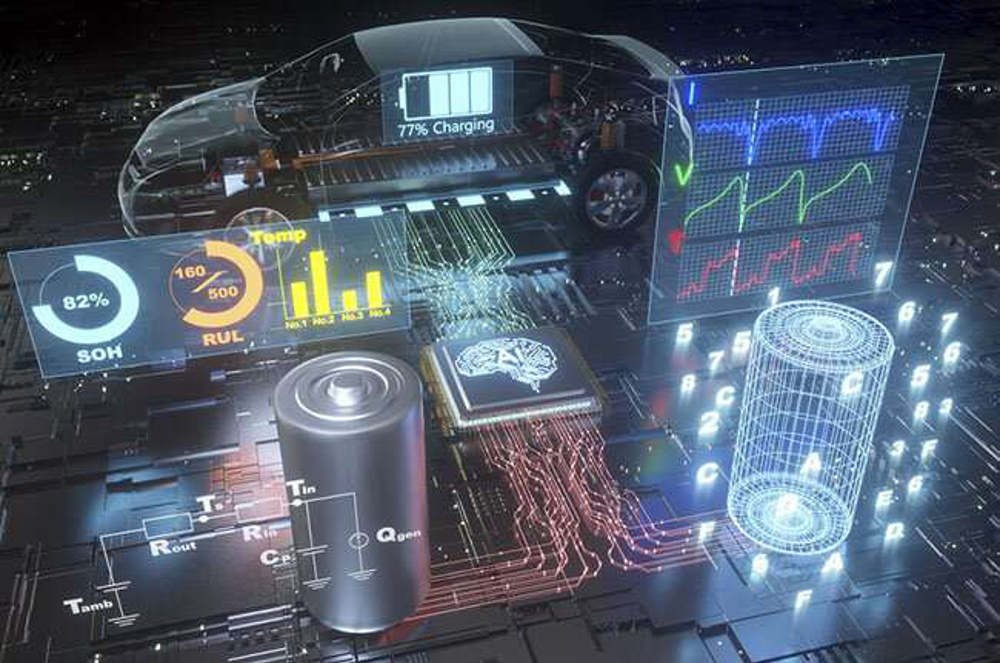
The methodology is analogous to running multiple experiments on each individual in a stadium full of "patients", and cross-checking with various control groups, all, unlike humans, totally unaware and unbiased to the experiments being conducted on them.
Using this approach, researchers can choose multiple experiments - each with 10 identical samples for statistical validation, as tests and controls - and let the machine learning algorithms 'learn' the group of batteries.
The genetic algorithm takes into consideration the hundreds of “genes” originating from chemistry formulations, cell design decisions, and production process measurements. Coupled with aggregated and augmented test measurements, a forest of decision trees can be created, dynamically, to explain the results.
This ad-hoc clustering method calculates the survivability of each battery (cell) in the resulting tree leaf and uses that information to maximize the pre-determined parameters. In essence, as the machine learns and improves the tree leaves - making the batteries more similar to each other – the better the lifetime predictions become.

The graph on the right is the survival rate of the selected nodes in the trees, where group number 2 with 105 subjects is represented in the graph as 2 in orange.
The resulting nodes in the trees are the algorithm's insights about how to improve battery life and can be used to establish cell design parameters, manufacturing parameters, or specific timed measurements.
Conclusion
Clearly, the benefit of AI and machine learning during the R&D process is proving invaluable in enabling researchers to evaluate the impact of changing more than one variable at a time, thereby speeding up the process.
However, this is not the only way in which AI can advance EVs. By embedding AI and machine learning into the EV's operational software it is possible to continuously monitor the performance and health of the battery, and by recording the data and learning from it, the battery's operational parameters can be updated in real-time.
At the same time, by creating smarter batteries with embedded sensing capabilities, and self-healing functionality, the battery management system can continuously monitor the 'state of health', and even rejuvenate selected battery cells or modules if required.
For the wide-scale adoption of EVs, the implementation of such AI and machine learning solutions could prove to be the key to overcoming one of the biggest consumer barriers - 'range anxiety'.
This article was first published in The AI Journal
Read more:
The role of AI in improving battery cell R&D productivity and speeding up the adoption of BEVs »